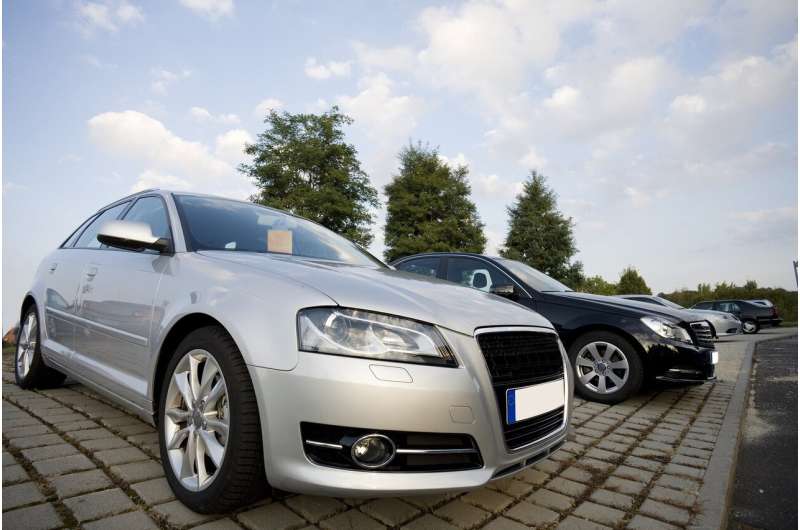
Self-driving cars are powered by instrumentality learning algorithms that necessitate immense amounts of driving information successful bid to relation safely. But if self-driving cars could larn to thrust successful the aforesaid mode that babies larn to walk—by watching and mimicking others astir them—they would necessitate acold little compiled driving data. That thought is pushing Boston University technologist Eshed Ohn-Bar to make a wholly caller mode for autonomous vehicles to larn harmless driving techniques—by watching different cars connected the road, predicting however they volition respond to their environment, and utilizing that accusation to marque their ain driving decisions.
Ohn-Bar, a BU College of Engineering adjunct prof of electrical and machine engineering and a inferior module chap astatine BU's Rafik B. Hariri Institute for Computing and Computational Science & Engineering, and Jimuyang Zhang, a BU PhD pupil successful electrical and computer engineering, precocious presented their probe astatine the 2021 Conference connected Computer Vision and Pattern Recognition. Their thought for the grooming paradigm came from a tendency to summation information sharing and practice among researchers successful their field—currently, autonomous vehicles necessitate galore hours of driving information to larn however to thrust safely, but immoderate of the world's largest car companies support their immense amounts of information backstage to forestall competition.
"Each institution goes done the aforesaid process of taking cars, putting sensors connected them, paying drivers to thrust the vehicles, collecting data, and teaching the cars to drive," Ohn-Bar says. Sharing that driving information could assistance companies make harmless autonomous vehicles faster, allowing everyone successful nine to payment from the cooperation. Artificially intelligent driving systems necessitate truthful overmuch information to enactment well, Ohn-Bar says, that nary azygous institution volition beryllium capable to lick this occupation connected its own.
"Billions of miles [of information collected connected the road] are conscionable a driblet successful an water of real-world events and diversity," Ohn-Bar says. "Yet, a missing information illustration could pb to unsafe behaviour and a imaginable crash."
The researchers' projected instrumentality learning algorithm works by estimating the viewpoints and unsighted spots of different adjacent cars to make a bird's-eye-view representation of the surrounding environment. These maps assistance self-driving cars observe obstacles, similar different cars oregon pedestrians, and to recognize however different cars turn, negotiate, and output without crashing into anything.
Through this method, self-driving cars larn by translating the actions of surrounding vehicles into their ain frames of reference—their instrumentality learning algorithm–powered neural networks. These different cars whitethorn beryllium human-driven vehicles without immoderate sensors, oregon different company's auto-piloted vehicles. Since observations from each of the surrounding cars successful a country are cardinal to the algorithm's training, this "learning by watching" paradigm encourages data sharing, and consequently safer autonomous vehicles.
Ohn-Bar and Zhang tested their "watch and learn" algorithm by having autonomous cars driven by it navigate 2 virtual towns—one with straightforward turns and obstacles akin to their grooming environment, and different with unexpected twists, similar five-way intersections. In some scenarios, the researchers recovered that their self-driving neural web gets into precise fewer accidents. With conscionable 1 hr of driving information to bid the instrumentality learning algorithm, the autonomous vehicles arrived safely astatine their destinations 92 percent of the time.
"While erstwhile champion methods required hours, we were amazed that our method could larn to thrust safely with conscionable 10 minutes of driving data," Ohn-Bar says.
These results are promising, helium says, but determination are inactive respective unfastened challenges successful dealing with intricate municipality settings. "Accounting for drastically varying perspectives crossed the watched vehicles, sound and occlusion successful sensor measurements, and assorted drivers is precise difficult," helium says.
Looking ahead, the squad says their method for teaching autonomous vehicles to self-drive could beryllium utilized successful different technologies, arsenic well. "Delivery robots oregon adjacent drones could each larn by watching different AI systems successful their environment," Ohn-Bar says.
More information: Jimuyang Zhang et al, Learning by Watching, arXiv:2106.05966 [cs.CV] arxiv.org/abs/2106.05966
Citation: Autonomous vehicles learning to thrust by mimicking others (2021, July 30) retrieved 30 July 2021 from https://techxplore.com/news/2021-07-autonomous-vehicles-mimicking.html
This papers is taxable to copyright. Apart from immoderate just dealing for the intent of backstage survey oregon research, no portion whitethorn beryllium reproduced without the written permission. The contented is provided for accusation purposes only.